5.1 Summary
R-Studio is a cross-platform disk recovery suite with stable, actively supported releases for Windows, Mac, and Linux. R-Studio for Windows, R-Studio for Mac, and R-Studio for Linux each deliver the same powerful disk recovery tools and user-friendly interface on their respective platforms. Version 5.0 builds on the already fast searching algorithms for FAT and NTFS partitions, it adds a new 'forensic mode' for the technician version, clickable links in the hexeditor and RAID 6 support. R-Studio can recover data from partitions, recover corrupted data and restore deleted files. Features and highlights. NAT/firewall traversal. R-Studio can recognize all RAID parameters for RAID 5 and 6. This feature helps the user to solve one of the most difficult problems in RAID recovery. Scan for Known File Types (raw file recovery): if the disk file system is heavily damaged or unsupported, R-Studio searches for specific data patterns ('file signatures') typical for certain file.
In this session, we’ll first learn how to read some external data (from .xls, .xlsx, and CSV files) into R with the readr
and readxl
packages (both part of the tidyverse
).
A new note I would like to share on some corner cases in expression evaluation in a few data processing languages (including R): My opinion on “5” 5 – Win Vector LLC. New York, NY 10018. From Business: About Studio ST Architects Studio ST Architects is a full-service, woman owned, architectural firm located in Manhattan that is dedicated to exploring the.
Then, we’ll write reproducible code to build graphs piece-by-piece. In Excel, graphs are made by manually selecting options - which, as we’ve discussed previously, may not be the best option for reproducibility. Also, if we haven’t built a graph with reproducible code, then we might not be able to easily recreate a graph or use that code again to make the same style graph with different data.
Using ggplot2
, the graphics package within the tidyverse
, we’ll write reproducible code to manually and thoughtfully build our graphs.
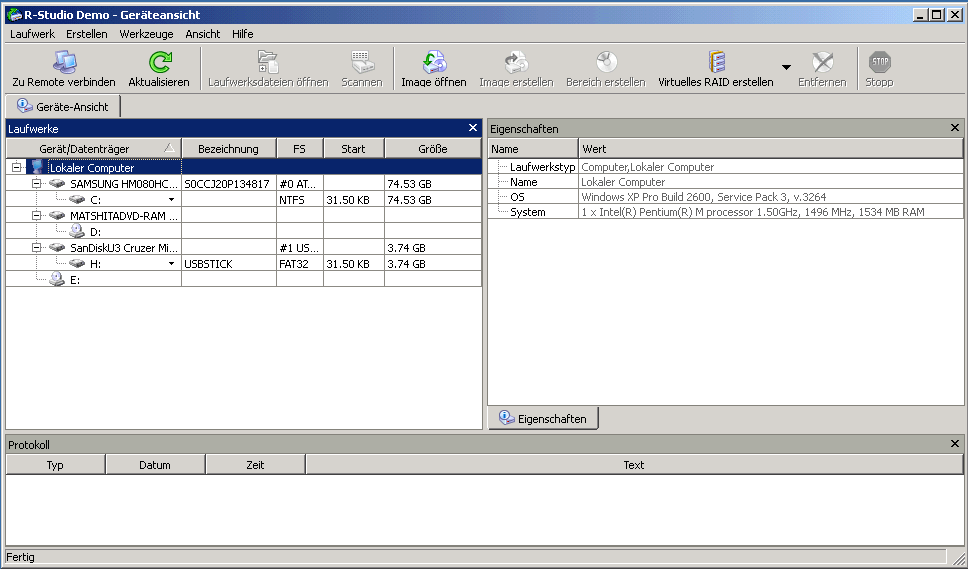
“ggplot2 implements the grammar of graphics, a coherent system for describing and building graphs. With ggplot2, you can do more faster by learning one system and applying it in many places.” - R4DS
So yeah…that gg
is from “grammar of graphics.”
We’ll use the ggplot2
package, but the function we use to initialize a graph will be ggplot
, which works best for data in tidy format (i.e., a column for every variable, and a row for every observation). Graphics with ggplot
are built step-by-step, adding new elements as layers with a plus sign (+
) between layers (note: this is different from the pipe operator, %>%
. Adding layers in this fashion allows for extensive flexibility and customization of plots.
5.1.1 Objectives
- Read in external data (Excel files, CSVs) with
readr
andreadxl
- Initial data exploration
- Build several common types of graphs (scatterplot, column, line) in ggplot2
- Customize gg-graph aesthetics (color, style, themes, etc.)
- Update axis labels and titles
- Combine compatible graph types (geoms)
- Build multiseries graphs
- Split up data into faceted graphs
- Export figures with
ggsave()
5.1.2 Resources
- readr documentation from tidyverse.org
- readxl documentation from tidyverse.org
- readxl workflows from tidyverse.org
- ggplot2-cheatsheet-2.1.pdf
- Graphs with ggplot2 - Cookbook for R
This lesson will show you how to load data, R Scripts, and packages to use in your Shiny apps. Along the way, you will build a sophisticated app that visualizes US Census data.
counties.rds
counties.rds
is a dataset of demographic data for each county in the United States, collected with the UScensus2010
R package. You can download it here.
Once you have the file,
R-studio 5.0
- Create a new folder named
data
in yourcensus-app
directory. - Move
counties.rds
into thedata
folder.
When you’re done, your census-app
folder should look like this.
The dataset in counties.rds
contains
- the name of each county in the United States
- the total population of the county
- the percent of residents in the county who are White, Black, Hispanic, or Asian
helpers.R
helpers.R
is an R script that can help you make choropleth maps, like the ones pictured above. A choropleth map is a map that uses color to display the regional variation of a variable. In our case, helpers.R
will create percent_map
, a function designed to map the data in counties.rds
. You can download helpers.R
here.
R-studio 5.4
helpers.R
uses the maps
and mapproj
packages in R. If you’ve never installed these packages before, you’ll need to do so before you make this app. Run Download avast free windows 10 64 bit.
R-studio 5.1
Save helpers.R
inside your census-app
directory, like below.
The percent_map
function in helpers.R
takes five arguments:
Argument | Input |
---|---|
var | a column vector from the counties.rds dataset |
color | any character string you see in the output of colors() |
legend.title | A character string to use as the title of the plot’s legend |
max | A parameter for controlling shade range (defaults to 100) |
min | A parameter for controlling shade range (defaults to 0) |
You can use percent_map
at the command line to plot the counties data as a choropleth map, like this.
Note: The code above assumes that census-app
is a sub-directory in your working directory. Make certain to set your working directory as the parent directory for census-app
. To change your working directory location, click on Session > Set Working Directory > Choose Directory… in the RStudio menu bar.
percent_map
plots the counties data as a choropleth map. Here it will plot the percent of white residents in the counties in the color dark green.
Loading files and file paths
Take a look at the above code. To use percent_map
, we first ran helpers.R
with the source
function, and then loaded counties.rds
with the readRDS
function. We also ran library(maps)
and library(mapproj)
.
You will need to ask Shiny to call the same functions before it uses percent_map
in your app, but how you write these functions will change. Both source
and readRDS
require a file path, and file paths do not behave the same way in a Shiny app as they do at the command line.
When Shiny runs the commands in server.R
, it will treat all file paths as if they begin in the same directory as server.R
. In other words, the directory that you save server.R
in will become the working directory of your Shiny app.
Since you saved helpers.R
in the same directory as server.R
, you can ask Shiny to load it with
Since you saved counties.rds
in a sub-directory (named data) of the directory that server.R
is in, you can load it with.
You can load the maps
and mapproj
packages in the normal way with
which does not require a file path.
Execution
Shiny will execute all of these commands if you place them in your app.R
script. However, where you place them will determine how many times they are run (or re-run), which will in turn affect the performance of your app, since Shiny will run some sections your app.R
script more often than others.
Shiny will run the whole script the first time you call runApp
. This causes Shiny to execute the server
function.
Shiny saves the server
function until a new user arrives. Each time a new user visits your app, Shiny runs the server
function again, one time. The function helps Shiny build a distinct set of reactive objects for each user.
As users interact with the widgets and change their values, Shiny will re-run the R expressions assigned to each reactive object that depend on a widget whose value was changed. If your user is very active, these expressions may be re-run many, many times a second.
Here’s what we’ve learned so far:
- The
shinyApp
function is run once, when you launch your app - The
server
function is run once each time a user visits your app - The R expressions inside
render*
functions are run many times. Shiny runs them once each time a user change the value of a widget.
How can you use this information?
Source scripts, load libraries, and read data sets at the beginning of app.R
outside of the server
function. Shiny will only run this code once, which is all you need to set your server up to run the R expressions contained in server
.
Define user specific objects inside server
function, but outside of any render*
calls. These would be objects that you think each user will need their own personal copy of. For example, an object that records the user’s session information. This code will be run once per user.
Only place code that Shiny must rerun to build an object inside of a render*
function. Shiny will rerun all of the code in a render*
chunk each time a user changes a widget mentioned in the chunk. This can be quite often.
You should generally avoid placing code inside a render
function that does not need to be there. Doing so will slow down the entire app.
Your Turn 1
Copy and paste the following app.R
file to your census-app
directory. Then add
to your app.R
script. Be sure to place the commands in an efficient location.
Note: This is the first of two steps that will complete your app. Choose the best place to insert the code above, but do not try to run the app. Your app will return an error until you replace # some arguments
with real code in Your Turn 2.
ui.R
Model Answer 1
Reveal answerSince your app only needs to load helpers.R
and counties.rds
once, they should go outside of the ui
and server
functions. This is also a good place to load the maps
library (which percent_map
uses).
You may wonder, “Won’t each user need their own copy of counties
and percent_map
?” (which would imply that the code should go inside of the server
function). No, each user will not.
Keep in mind that your user’s computer won’t run any of the R code in your Shiny app. In fact, their computer won’t even see the R code. The computer that you use as a server will run all of the R code necessary for all of your users. It will send the results over to your users as HTML elements.
Your server can rely on a single global copy of counties.rds
and percent_map
to do all of the R execution necessary for all of the users. You only need to build a separate object for each user if the objects will have different values for each of your users.
Finishing the app
The census visualization app has one reactive object, a plot named 'map'
. The plot is built with the percent_map
function, which takes five arguments.
R Studio 5.1 Download
- The first three arguments,
var
,color
, andlegend.title
, depend on the value of the select box widget. - The last two arguments,
max
andmin
, should be the max and min values of the slider bar widget.
The server
function below shows one way to craft reactive arguments for percent_map
. R’s switch
function can transform the output of a select box widget to whatever you like. However, the script is incomplete. It does not provide values for color
, legend.title
, max
, or min
. Note: the script will not run as is. You will need to finish the script before you run it, which is the task of Your Turn 2.
Your Turn 2
Download windows 10 using utorrent. Complete the code to build a working census visualization app.
When you’re ready to deploy your app, save your app.R
file and run runApp('census-app')
. If everything works, your app should look like the picture below.
You’ll need to decide
- how to create the argument values for
percent_map
, and - where to put the code that creates these arguments.
Remember, you’ll want the argument values to switch whenever a user changes the associated widget. When you are finished, or if you get stuck, read on below for a model answer.
Model Answers 2
Recap
You can create more complicated Shiny apps by loading R Scripts, packages, and data sets.
5 Number Summary Rstudio
Keep in mind:
- The directory that
app.R
appears in will become the working directory of the Shiny app - Shiny will run code placed at the start of
app.R
, before theserver
function, only once during the life of the app. - Shiny will run code placed inside
server
function multiple times, which can slow down the app.
You also learned that switch
is a useful companion to multiple choice Shiny widgets. Use switch
to change the values of a widget into R expressions.
As your apps become more complex, they can become inefficient and slow. Cc2540 driver windows 7. Lesson 6 will show you how to build fast, modular apps with reactive expressions.
If you have questions about this article or would like to discuss ideas presented here, please post on RStudio Community. Our developers monitor these forums and answer questions periodically. See help for more help with all things Shiny.